
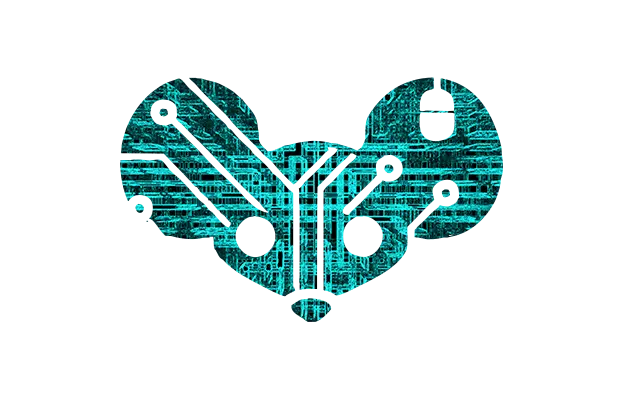
I think what they meant by that is “is this different wrt antitrust compared to Intel and x86?”
Intel both owns the x86 ISA and designs processors for it, though the situation is more favorable in that AMD owns x86-64 and obviously also designs their own processors.
I’m fairly certain blockchain GPUs have very different requirements than those used for ML, especially not LLMs. In particular they don’t need anywhere as much VRAM and generally don’t require floating point math, nor do they need features like tensor cores. Those “blockchain GPUs” likely didn’t turn into ML GPUs.
ML has been around for a long time. People have been using GPUs in ML since AlexNet in 2012, not just after blockchain hype started to die down.